Penn State National Science Foundation Center for Health Organization Transformation
(Penn State CHOT) - An Industry-University Cooperative Research Center
|
Wishing you all a happy fall
Pattee Library and Paterno Library on Penn State's University Park campus, Sept 22, 2020.
Photo credit: Haedong Kim
|
Christopher DeFlitch featured on WPSU's Conversation Live
Christopher DeFlitch, Penn State Health vice president and chief medical information officer and CHOT co-principal investigator, discussed critical topics regarding the COVID-19 pandemic with guests Niki vonLockette, associate professor of public policy and African American studies, and Beth McGraw, professor and Huck Scholar in Entomology. They talked about how much longer the pandemic will last, when we will get a vaccine, how people of color have been disproportionately impacted, and more.
|
Penn State CHOT Research Highlights
|
|
Spatiotemporal Regularization for Inverse ECG Modeling
Bing Yao and Hui Yang developed a novel spatiotemporal regularization method for inverse electrocardiographic (ECG) modeling. Advanced sensing such as the wearable sensor network provides an unprecedented opportunity to capture the wealth of information pertinent to space-time electrical activity of the heart, and facilitate the inverse ECG modeling with the readily available data of body surface potential mapping. However, it is often challenging to derive heart-surface potentials from body-surface measurements, which is called the "inverse ECG problem." Traditional regression is not concerned about spatiotemporal dynamic variables in complex geometries, and tends to be limited in the ability to handle high-dimensional spatiotemporal data for solving the inverse ECG problem.
This paper presents a comparison study of regularization methods in the performance to achieve robust solutions of the inverse ECG problem. The researchers first introduce the forward and inverse ECG problems. Second, the researchers propose two spatiotemporal regularization (STRE) models to increase the robustness of inverse ECG modeling. Finally, case studies are conducted on the two-sphere geometry, as well as a real-world torso-heart geometry to evaluate the performance of different regularization methods. Experimental results show that STRE models effectively tackle the ill-conditioned inverse ECG problem and yield 56.3% and 67.3% performance improvement compared to the traditional Tikhonov regularization in the two-sphere and the torso-heart geometries, respectively. The spatiotemporal regularization methodology is shown to have strong potential to achieve robust solutions for high-dimensional predictive modeling in the inverse ECG problem.
This work is published in IISE Transactions on Healthcare Systems Engineering and is available on Taylor & Francis Online. DOI: 10.1080/24725579.2020.1823531 |
Full texts are available online for the five papers accepted to the
IEEE Engineering in Medicine and Biology Conference (EMBC) 2020.
In a previous newsletter, we shared that five papers by CHOT scholars were accepted to IEEE EMBC 2020. They are now available online. In response to the coronavirus pandemic, EMBC was held virtually in July. Click here to watch the presentations by the authors.
1. Dynamic Physician-Patient Matching in the Health Care System
Ruimin Chen developed a novel dynamic physician-patient matching algorithm. Long patient waiting times has attracted the public's attention significantly due to the negative effects on patients' satisfaction with health systems in the United States. Due to the ineffective allocation between physicians and patients, the growing population needing health care, and the restriction introduced by insurance policies, the waiting time of a patient to see a physician for the first time has been increased by 30% since 2014. There is an urgent need to develop matching mechanisms with the consideration of preferences from both patients and physicians for improving matching results. This paper presents a new allocation framework between physicians and patients to shorten the patient waiting time as well as improve the allocation effectiveness. We leverage the matching theory and extend the conventional deferred acceptance algorithm to a discrete-time stable marriage framework (i.e., discrete deferred acceptance algorithm, DDA) with the consideration of uncertainty constraints introduced by insurance types. The proposed algorithm is benchmarked against the current practice (i.e., continuous deferred acceptance scheme, CDA) under different scenarios when the demand-supply ratio (DSR) varies. Experimental results show that when the DSR is more than 1.25, DDA outperforms traditional CDA practices in terms of waiting time and matching regret. The proposed framework shows strong potential to tackle the problem of long waiting times in the health care system in America.
2. Markov Decision Process Modeling for Multi-stage Optimization of Intervention and Treatment Strategies in Breast Cancer DOI: 10.1109/EMBC44109.2020.9175905
Farhad Imani developed a novel method based on Markov decision process modeling for interventions and treatment strategies in breast cancer. Breast cancer is a prevalent problem that undermines quality of patients' lives and causes significant impacts on psycho-social wellness. Advanced sensing provides unprecedented opportunities to develop smart cancer care. The available sensing data captured from individuals enable the extraction of information pertinent to the breast cancer conditions to construct efficient and personalized intervention and treatment strategies. This research develops a novel sequential decision-making framework to determine optimal intervention and treatment planning for breast cancer patients. This paper proposes a Markov decision process (MDP) model for both objectives of intervention and treatment as well as quality adjusted life years (QALYs) with the data-driven and state-dependent intervention and treatment actions. The state space is defined as a vector of age, health status, prior intervention, and treatment plans. Also, the action space includes wait, prophylactic surgery, radiation therapy, chemotherapy, and their combinations. Experimental results demonstrate that prophylactic mastectomy or chemotherapy is more effective in minimizing the expected cancer cost of 25-to-60-year-old patients with in situ stage of cancer. However, wait policy leads to an optimal quality of life for a patient with the same condition. The proposed MDP framework can also be generally applicable to a variety of medical domains that entail evidence-based decision making.
3. Statistical Analysis of County-Level Contributing Factors to Opioid-Related Overdose Deaths in the United States DOI: 10.1109/EMBC44109.2020.9176465
Haedong Kim applied a statistical method to investigate contributing factors to opioid overdose deaths. To be specific, this paper examines county-level characteristic factors contributing to opioid-related overdose deaths in the United States. The authors categorized factors into three groups: demographic, socio-economic, and health care environmental group. These features were used as predictors to model the overdose deaths from all types of opioids including prescription (e.g., oxycodone and hydrocodone) and illicit opioids (e.g., heroin and fentanyl) to investigate general trends, as well as separate models for heroin and fentanyl. Multilevel mixed-effect regression was adopted to adequately model grouping effect across counties.
4. Gradient Mechanism to Preserve Differential Privacy and Deter Against Model Inversion Attacks in Health Care Analytics DOI: 10.1109/EMBC44109.2020.9176834
Alexander Krall developed a novel gradient mechanism to maintain differential privacy while also mitigating the risk of model inversion attacks in health care information systems. Advanced sensing technologies, driven by the Internet of Things, have caused a sharp increase in data availability within the health care system. The newfound availability of data offers an unprecedented opportunity to develop new analytical methods to improve the quality of patient care. Data availability, however, is a double-edged sword. Malicious attacks and data breaches are increasingly seen in the health care field, which result in costly disruptions to operations. Adversaries exploit analytic models to infer participation in a dataset or estimate sensitivity attributes about a target patient. This paper is aimed at developing a differentially private gradient-based mechanism and assessing its utility in mitigating the impact of these attack risks within the context of the intensive care units. Experimental results showed that this methodology is capable of greatly reducing the risk of model inversion while retaining model accuracy. Thus, health systems that employ this technique can be given more peace of mind that high-quality services can be delivered in such a way that privacy is preserved.
5. Hierarchical Gaussian Process Modeling and Estimation of State-action Transition Dynamics in Breast Cancer DOI: 10.1109/EMBC44109.2020.9175984
Zihang Qiu developed a novel hierarchical Gaussian process-based method to model and estimate state-action transitions in breast cancer. Breast cancer is the most common type of cancer among women in the U.S. Available treatments, including mastectomy, radiation, and chemotherapy vary in curability, cost, and mortality probability of patients. This research aims at tracking the result of post-treatment for evidence-based decision making in breast cancer. Based on available big data, the researchers implemented conditional probability to estimate multi-age transition probability matrices to predict the progression of disease conditions. The patient state is defined based on patients' age, cancer stage, and treatment history. To tackle the incomplete data in the matrix, we designed a novel Hierarchical Gaussian Distribution (HGP) to estimate the missing part of the table. The HGP model leads to the lowest Root Mean Square Error (RMSE), which is 35% lower than Gaussian Process and 40% lower than Linear Regression. Results of transition probability estimation show that the chance of survival within a year for 40-to-50-year old patients with the distant stage of cancer is 96.5%, which is higher than even younger age groups.
|
Adhithya Padmanabhan and Cameron Butts
| 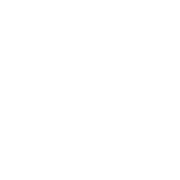 | Adhithya | 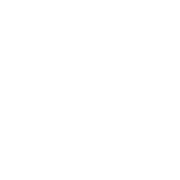 |
| 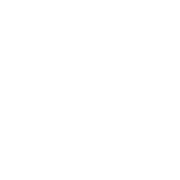 | Cameron | 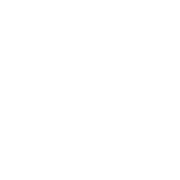 |
Adhithya Padmanabhan, a second-year Ph.D. student in transportation engineering, joined CHOT in fall 2020. He is working on the interrelationships among transportation networks, human traffic, and the spread of COVID-19 in the U.S. The world has seen drastic changes since the COVID-19 pandemic began, and there has been extensive research done to study the factors affecting its spread. However, very little has been done to investigate how human interactions in the transportation network affect the spread of the virus in different counties in the U.S. By developing a pattern and understanding the characteristics of the transportation network, this research aims to forecast the further spread of the disease and such diseases in the future.
Cameron Butts, an engineering student at Penn State Behrend, also joined CHOT in fall 2020. He is working with CHOT faculty member Faisal Aqlan to develop a virtual reality system to improve health care experiences of patients.
Welcome, Adhithya and Cameron!
|
As event guidance concerning the COVID-19 pandemic continues to evolve, please note that these events may be rescheduled or moved to a virtual venue. Please check in with the specific event planners for the latest information.
CHOT Fall 2020 IAB Meeting
Oct. 2 from 1:00 p.m. CST to 3:30 p.m. CST
In response to the spread of COVID-19, the increasing travel restrictions, and for the health and safety of all our members, faculty, and students, the CHOT leadership team has decided to host the Fall IAB meeting virtually. The fall conference call will be held on Friday, Oct. 2 from 1:00 p.m. CST to 3:30 p.m. CST in order to discuss proposals and additional CHOT business. Click here to register.
IISE Annual Conference 2020
Oct. 31-Nov. 3
New Orleans, Louisiana
In response to the COVID-19 pandemic, the IISE Annual Conference & Expo has been rescheduled from May 30-June 2 to Oct. 31-Nov. 3 in New Orleans, Louisiana at the Hyatt Regency New Orleans. Leaders in the field will join up-and-comers and students to network, gather new ideas, and learn about innovative tools and techniques - resulting in lasting career connections. Please check the revised schedule and new registration dates. Click here for more information.
|
Check out the CHOT website for information about our new projects and exciting activities.
|
If you have any exciting news that you would like to feature in our CHOT newsletter, please submit it to Haedong Kim, newsletter editor, at [email protected].
|
|